Sentiment Analysis using Data Scrapping
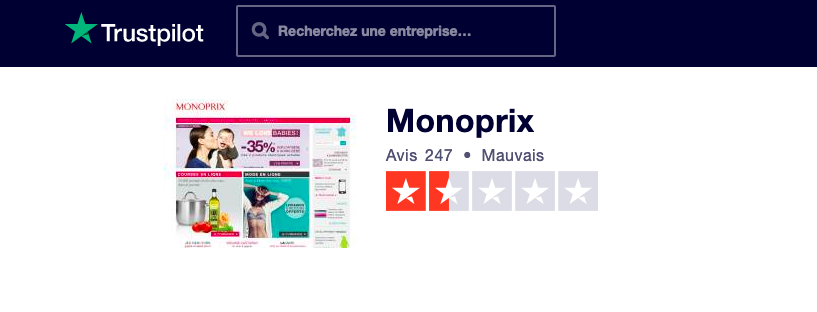
Automating Qualitative Market Research
I have recently been criticized for spending much of my focus on strategic marketing while ignoring online marketing completely. It’s a fair point; indeed, my background makes me show strong preference for things like product and brand positioning or customer segmentation. Another reason why I didn’t dedicate time to mundane tasks like recommender systems or text mining is that there’s already lots of information available on these subjects. Anyway, today I’ll make up for this and will illustrate how modern data science algorithms can be helpful for community managers and people involved in online marketing, not just marketing managers.
First, I’ll show how data can be scrapped from online resources with example of Trustpilot reviews. I will then walk you through text mining to illustrate how the meaningful insights can be extracted from text. Finally, I will apply few modern machine learning techniques to predict if the review is positive or negative.
As a word of caution, I am not going to use the vastly developed and complex NLP techniques for text analysis. First, because I wanted to avoid this immensely broad topic, but mainly because I wanted to illustate how supposedly simple techniques like bag-of-words and frequency tables are still very powerful and largely sufficient for most applications.
Finally, in sentiment analysis we normally talk about English text, English dictionaries. Sentiment analysis in other languages is a much less known field. I enjoyed discovering dictionaries for French text mining and I’m impatient to share my findings.
Data scrapping
For the purpose of this exercise I’ve accessed Trustpilot reviews of Monoprix. This is what the page looks like:
As you can see, the reviews are spread through several pages, so I write a small script that goes through all fo them, collecting data. To access the elements that are of interest, I look at the souce code of the page first.
For instance, I want to collect the review title, text and the rating. That’s what the code for the rating looks like:
rank_data <- webpage %>%
html_nodes('.star-rating img') %>%
html_attr("alt")
As a result we have the following dataset with 234 observations (reviews):
Obviously, the description text is rough, has lots of special characters and needs a through cleaning before sentiment analysis can be run on it.
Text mining and preliminary analysis
I convert the review text into a special object called text corpus and run transformations on it. The basic ones are removing numbers, punctuation, extra white space and special characters like apostrophes and semicolons, followed by transforming the text to lower case.
Next, the stopwords are removed (the words that are common to a given language but don’t give out any sentiment, like, for instance, ‘is’, ‘are’ and ‘the’ in English or ‘de’, ‘la’ and ‘sont’ in French).
This is what the stripped text looks like:
## <<SimpleCorpus>>
## Metadata: corpus specific: 1, document level (indexed): 0
## Content: documents: 4
##
## [1] moitié produits commandés ligne disponibles donc livrés livraison a lieux minutes plus tard heure indiqué fruit légumes sûrement pires stock plus tout produits date expiration lendemain jours suivants!plus jamais
## [2] pourquoi monoprix embauche seniors cv jamais entretien
## [3] bonjour souhaite souligner efficacité service client ainsi gentillesse disponibilité personnel aime acheter articles mode ainsi lingerie excellent rapport qualité prix côté très branché plus période soldes beaucoup affaires disponibles compris site bravo
## [4] monoprix narbonne a tres evolué espaces fonctionnels tres organisés tres jolies choses tres pratiquepar contre responsable tres désagréable repond sèchement moque clients certaines salariées aucune discretion dommage peut etre direction pourrait envoyer formation puisse acquérir certaines bases accueil respect clientcela plus ans viens magasin bien plus venais mere ponpon
Finally, the text corpus is sometimes stemmed. Stemming is removing the words endings so that the root only counts for sentiment analysis. In the stemmed text, the words ‘run’, ‘runs’ and ‘running’ will count as the same word three times. It makes sense for machine analysis, but doesn’t please to a human reader. Thus, I skip stemming and move on to building a term document matrix, which is basically a matrix containing the frequency of the terms we encounter in the analyzed text.
Very commonly, it is a sparse matrix, meaning that the object is large, but most of the words are only there once or twice. For the ease of manipulating the data and also because these rare words are not of any use, the sparse terms are removed from the matrix.
So, below are the Top 10 common words that are seen in Monoprix reviews on Trustpilot:
Visually it could be shown in the histogram form:
However, the most common representation is the wordcloud, which is basically just an infographic where the words’ size correspond to their frequency in the text.
While this is a nice representation, undobtedly, it is not very useful on its own. However, it can give the ideas where to explore further. For example, we can see the associated terms with each of the commonly shown terms (the number next to each term is the correlation level).
service | |
---|---|
client | 0.55 |
réponse | 0.48 |
toujours | 0.42 |
nouvelle | 0.38 |
nouveau | 0.38 |
personne | 0.37 |
aucune | 0.34 |
part | 0.34 |
demande | 0.32 |
jamais | |
---|---|
plus | 0.3 |
juste | 0.29 |
manque | 0.27 |
internet | 0.25 |
horaire | 0.24 |
livraison | 0.21 |
bon | 0.21 |
livraisons | 0.21 |
aucun | |
---|---|
nouveau | 0.37 |
respect | 0.34 |
cher | |
---|---|
livraisons | 0.33 |
plus | 0.26 |
The applications of term associations are more interesting. For example, as a consultant hired to improve marketing management, and without any prior knowledge about Monoprix and its problems I can quickly come up with the conclusion that there are underlying problems with missing articles when groceries are delivered, as well as some problems with on-time delivery. It seems that the reviewing customers no longer want to use internet to order groceries. It might also be that the customers perceive the groceries ordered online as more expensive.
Of course, all these findings are preliminary and need somebody looking forward into them. They should not be presented to the board of directors as the cause of the customer dissatisfaction. However, it is a useful basis for data collection and investigation.
Sentiment Analysis
The most ancient and robust technique of sentiment analysis is based on taking the so-called dictionaries (the list of positive and negative words for the given language) and counting the number of instances, or rather, the percentage, for each of the words. For instance, if the first review has 30% negative words and 10% of positive words (because some words are, obviously, neutral), then we might consider that the review will most likely have a negative ranking (one or two stars).
This approach has a number of flaws. One of them is the treatment of negation and another is the question: what do you consider positive and negative? Is ‘milk’ considered positive? It might in some context (ask your toddler!), but if we’re reading supermarket reviews about groceries, it is most likely neutral.
What about the figures of speach? What about building the dictionaries for every language?
Despite these inconveniences, the method is still highly effective, as I’m going to demonstrate. To do so, I will use the FEEL dictionary (FEEL: French Expanded Emotion Lexicon. Special thanks to Amine Abdaoui, Jérôme Azé, Sandra Bringay et Pascal Poncelet).
Below is the list of the top review words that were considered positive, along with the supposedly negative words.
Positive: heure, lendemain, ligne, livraison, client, personnel, qualité, service, site, accueil, bien, magasin, commande, temps, enseigne, bon, message, article, réponse, jour, achat, bref, carte, compte, domicile, expérience, montant, caisse, personne, rayon, aller, mettre, panier, surtout, créneau, horaire, produit, part and suite
Negative: dommage, moins, mal, problème, cher, appel, retard and déplorable
We will see further down that even if some of the positive words don’t have real positive connotation, it doesn’t have much impact on the accuracy of the analysis.
We will combine the words and their (relative, in %) frequencies in the following dataframe, where each row correspond to the review, so there’s 234 rows in total:
Note that the last column is the actual ranking coming from the rating in stars, that was converted for simplicity into zero (negative review) or one (positive review). The reason for this binary conversion is that we have a small amount of data (234 reviews), and the majority of them are very negative. It will be difficult or nearly impossible to teach the algorithm to distinguish between 4- and 5-stars reviews if there aren’t any or if there’s very little of those.
Prediting a positive or negative review
We’re going to split 234 reviews into a training and test set, based on 70%/30% rule. We’ll train the algorithm on training set and evaluate its prediction on the test set, the data the algorithm hasn’t yet seen.
Support Vector Machines
We’ll start with Support Vector Machines algorithm, tune it, and use it for prediction.
By looking at the confusion matrix we see that the algorithm is doing an okay job predicting bad reviews, but completely fails to identify good ones, throwing them all into the same class.
The Area under ROC curve is 0.62, meaning that the prediction is only slighly better than the random guess (0.5).
Random Forests
Random Forest is doing a much better job, correctly identifying around 93% of the reviews.
Naive Bayes
We’ll also try Naive Bayes classification that shows similar performance to SVM, mostly miss-classifying the positive reviews, as they are scarce in the data to learn from.
Neural Networks (NNet)
Next, we’ll train the neural network from NNet package. It is a relatively rigid package that allows building a network with BFGS algorithm only, and only using a sigmoid function for hidden layer activation. There’s only one hidden layer that we choose to build with 35 nodes.
Despite a relative simplicity of this network, it does quite a good job classification job and we correctly predict over 92% of the review rankings.
ANN with Keras and Tensorflow
Finally, we will use a well-known Keras package that provides interface to Tensorflow, in order to build a deep learning neural network with two hidden layers (40 nodes each), sigmoid activation and adam optimizer. We train it with validation split 80%/20% and end up with a pretty good accuracy.
I daresay that I was slighly dissapointed with Keras performance compared to Nnet performance. It’s not that it wasn’t splendid, it’s just that all the fuss around it makes us thinks that it works wonders.
In fact, this example shows once again that no algorithm on its own is better than another; it all depends on the choice of the algorithm for a given problem, parameters tuning, etc.
Overall, we notice that NNet network shows better accuracy while Keras network behaves slighly better in terms of area under ROC metric.
And, finally, the summary of all models’ performance.
SVM | Random Forest | Naive Bayes | NNet | Keras | |
---|---|---|---|---|---|
In Sample Accuracy | 0.87 | 0.83 | 0.84 | 0.86 | 1 |
Out Sample Accuracy | 0.86 | 0.94 | 0.86 | 0.93 | 0.88 |
Total Accuracy | 0.86 | 0.94 | 0.86 | 0.93 | 0.88 |
In Sample Precision | 0.87 | 0.88 | 0.87 | 0.89 | 1 |
Out SamplePrecision | 0.86 | 0.88 | 0.88 | 0.95 | NA |
Total Precision | 0.86 | 0.95 | 0.87 | 0.93 | 0.89 |
In Sample Recall | 1 | 0.93 | 0.97 | 0.95 | 1 |
Out Sample Recall | 1 | 0.94 | 0.99 | 1 | NA |
Total Recall | 1 | 0.98 | 0.99 | 0.99 | 0.99 |
To summarize, even on this very imperfect dataset with a small number of obervations and little reviews belonging to one of the classes, the models are able to make quite a good prediction. From business point of view, it means that we don’t need to make a huge effort in order to identify the sentiment of the customer reviews correctly. Automating this task helps keeping an eye on community and quickly identifying the areas where human interaction is needed.
Today, qualitative marketing can be largely automated using data scrapping and text mining methods. Deploying these methods takes relatively little time, but allows for a huge time-saving for marketers and community managers.