Blog - Business Data Science
January 22nd
SNCF Stations Clustering
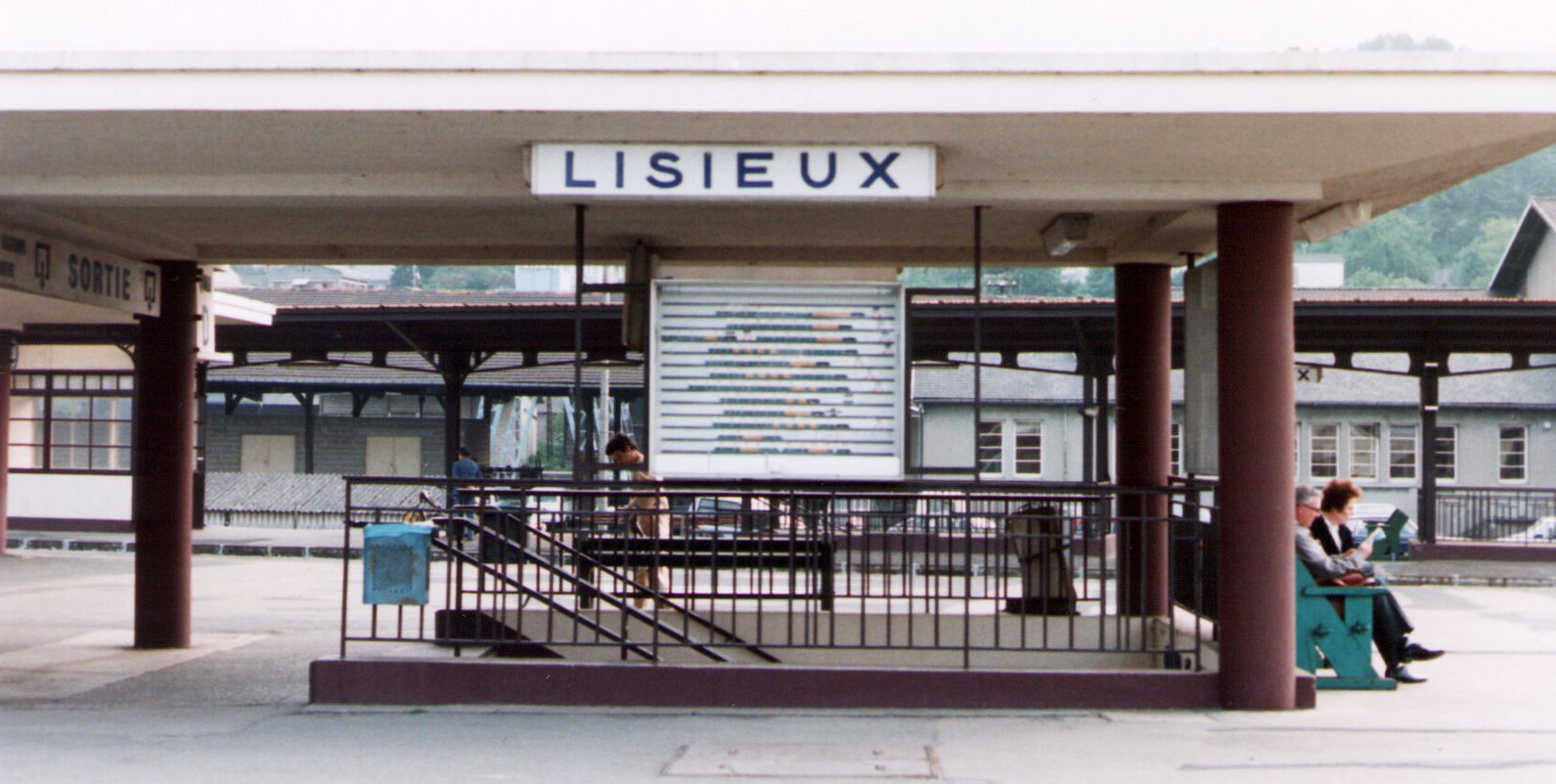
This time I dissect SNCF customer satisfaction survey to find latent variables that have the most impact on customer satisfaction. These findings show the areas of improvement where potential investment could be made.
Next, by applying hierarchical clustering algoritms, I succeed in obtaining meaningful clusters of train stations. I show how these clusters compare and where customer satisfaction fits in this big picture.
January 7th
SNCF Satisfaction Survey

Using SNCF customer satisfaction survey available thanks to their Open Data initiative, I illustrate how Data Science can be important for managerial decisions. The unexpected findings that I uncover surprised me just as much as they could surprise SNCF decision-makers.
Customer satisfaction in SNCF train stations can be easily and quite accurately predicted based on very few prediction variables. Various models exhibit similar prediction quality, confirming our findings that despite fancy marketing moves, some costly investments into station amenities don't play an important role in customer satisfaction.
January 1st
Data Science Challenge
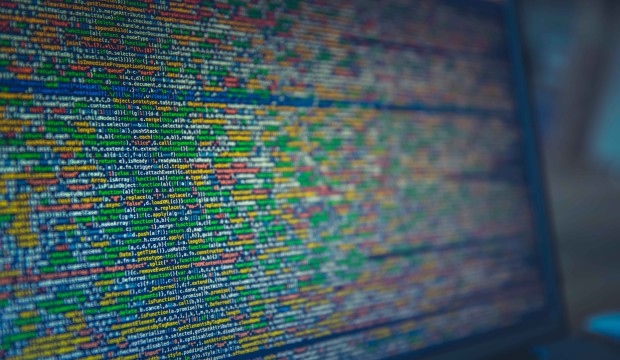
The main challenge of any simple project is a vast amount of different files that had to be cleaned and combined together before the data analysis could even start. Data preparation is one of the unseen parts of Data Scientist’s job, this hidden part of the iceberg.
I have recently looked into publicly available SNCF customer satisfaction survey. My goal was to illustrate how Data Science can be important for such simple managerial decisions as investment in simple amenities. I used this opportunity to show how tedious the simple task of data manuipulation can be.
December 20th
Supermarkets: the only differentiator is price
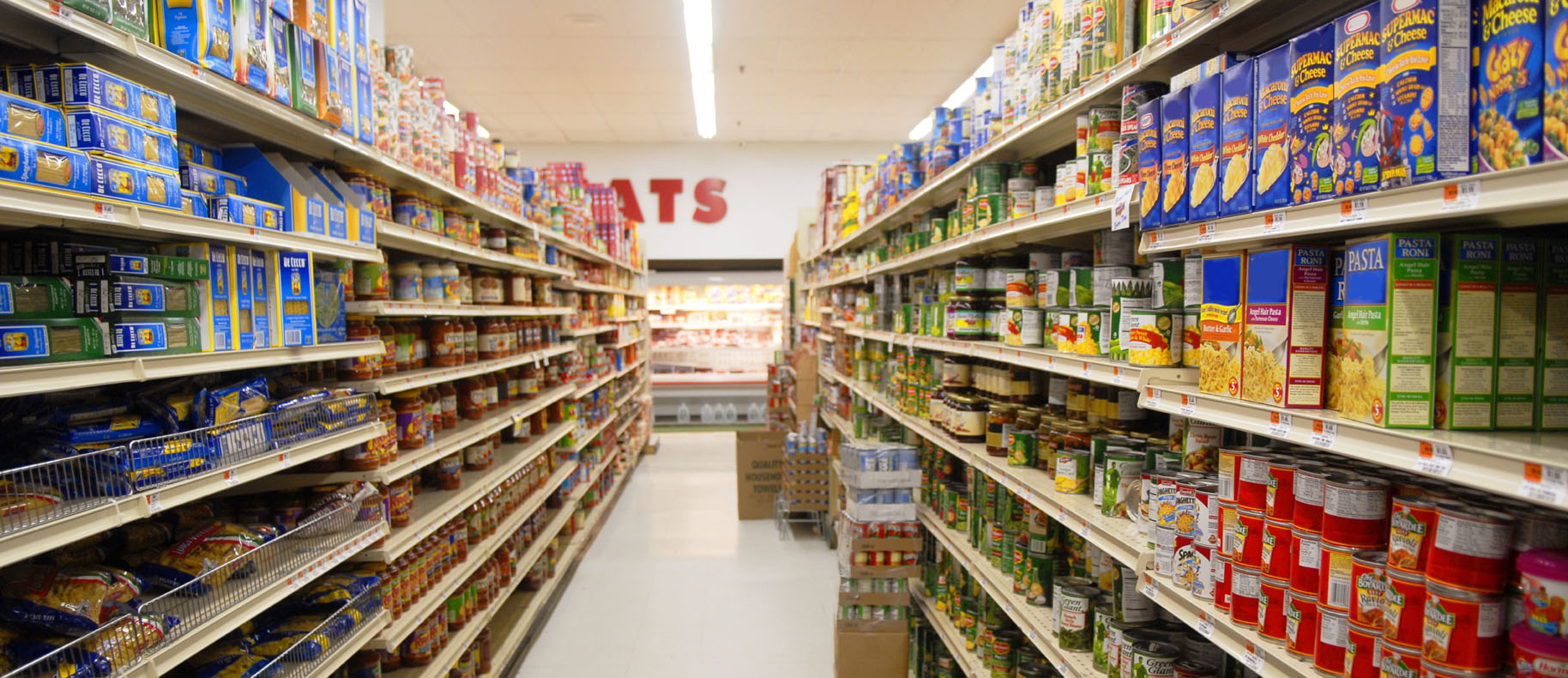
Some leading marketers speak today of "personalized mass consumption": the increasing demand for quality and variety of products that causes a change in the nature of mass consumption. This trend, together with the slowdown in growth "by sales area" has turned differentiation into a necessity. Price-centered differentiation strategy was greatly weakened by Galland law (1996), that pushed to reduce the price differences between brands on national brand products.
Without giving up on discounting, supermarkets today are adopting a more qualitative differentiation strategy, the objective of which is to build customer loyalty. Exploratory Factor Analysis show the latent customer perceptions about different brands and helps us see how different supermarkets brands are differentiated.
December 9th
Monoprix is expensive, but otherwise indifferentiated
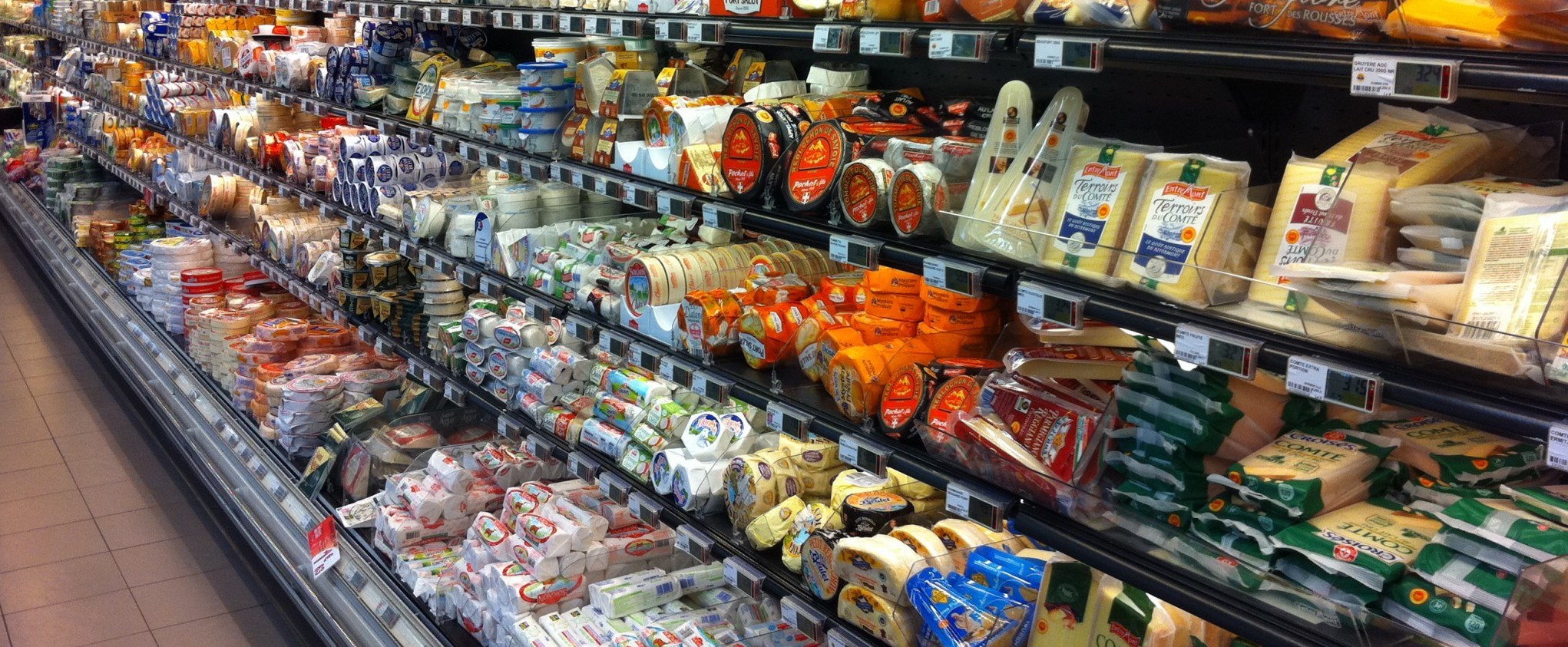
The competition in the mature commodity market with very little margin for product differentiation (groceries) is tight. The big brands pursue extensive growth by increasing the store surfaces and establishing presence. It is a question of better adapting to the expectations of the clientele, by combining price undercutting (« lutte contre la vie chère ») and qualitative differentiation, such as CSA, for instance. Some brands have more success than others: let's investigate.
Using the customer brand perception survey, I interpret the brand-related adjectives and keywords noted on Likert scale. Applying PCA (Principal Components Analysis, a common Data Science dimensinality-reduction tool), I extract the dimensions to plot the data against, in 2D. The result is perceptual map that allows assessing brand positioning and differentiation.
November 9th
Automating Qualitative Market Research
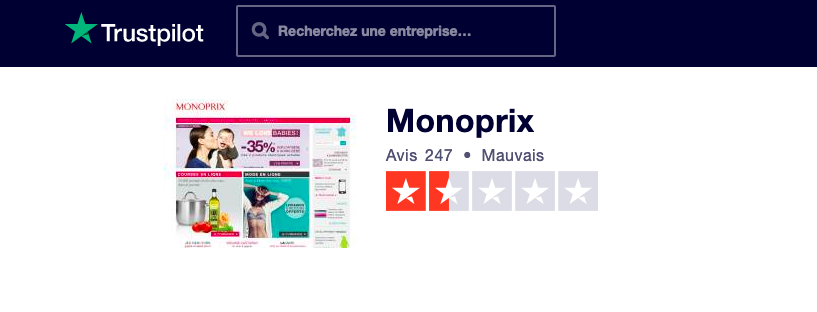
Today, qualitative marketing can be largely automated using data scrapping and text mining methods. Deploying these methods takes relatively little time, but allows for a huge time-saving for marketers and community managers.
I illustrate data scrapping by collecting Monoprix reviews from Trustpilot. The data mining and sentiment analysis are performed using bag-of-words and word-frequency matrix approach. Finally, I predict if the review is positive or negative using several most popular Data Science methods, including deep learning with Tensorflow.